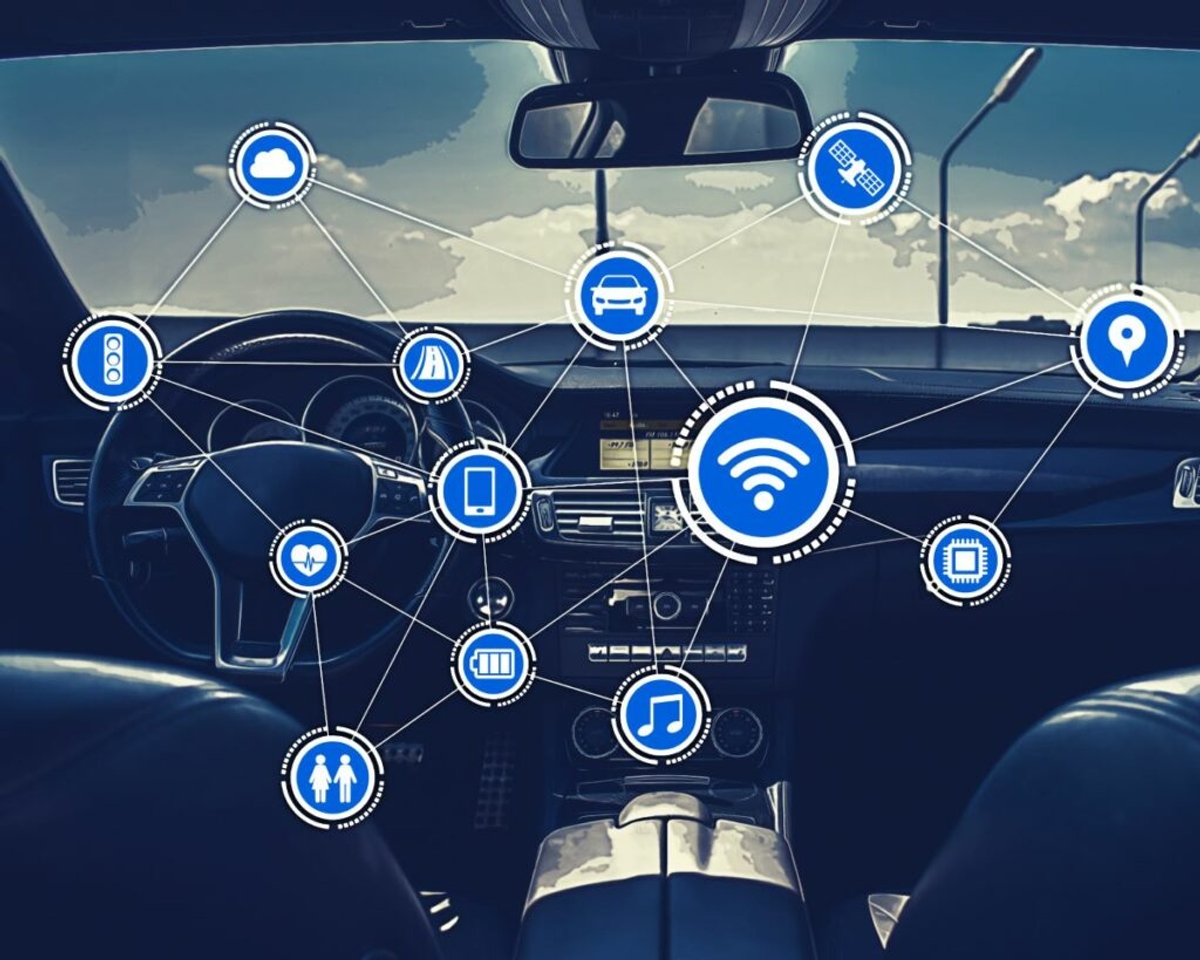
Most modern cars do not use all the driverless technology available much like Automated Marking and Natural Language Processing tools
What is Automated Marking?
Let’s start by trying to clarify what is a complicated area of computing and assessment.
Automated Marking systems are built using Large Language Models (LLMs) like the ones underpinning ’generative’ AI such as ChatGPT.
However, because these conversational models are built with such a broad range of information – some that will be out of date or plain wrong – they generate human-like responses that seem plausible but may be incorrect or irrelevant.
Automated Marking (AM), on the other hand, uses a ‘predictive’ AI aimed at far greater accuracy.
Researchers building AM use thousands of relevant student responses to specific assessment items to fill an AI framework for marking further work from across the grade spectrum. Markers notes and mark schemes could also be added.
The AM identifies language patterns and features associated with different levels of subject proficiency.
From this it could analyse and score unmarked writing.
The strengths of Automated Marking
It would be as if a human has memorised the work of a panel of the subject’s top examiners.
In addition, they have instant recall of all the scripts and mark schemes and, to top it off, can use that information to process a paper in seconds.
No human could match AM for speed.
And Automated Marking's weaknesses?
Conversely, there are some things humans can do which machines can’t.
Auto-markers are not great for nuanced marking above functional level subject assessment.
Experienced markers, unlike AM, can distinguish between a long jumble of relevant jargon and a coherent, logical and accurate open form answer.
This relative unsophistication means we are not looking to replace humans with AM but rather to assist them.
So what should we do with Automated Marking?
A good analogy for Automated Marking are driverless cars.
Technology exists to replace drivers' feet, hands and eyes, but currently it struggles with split-second ethical decisions humans face while driving.
The accountability chain in accidents involving AI also becomes complicated.
This is why the adoption of driverless technology more often than not focuses on smaller features like Lane Assist or Automated Parking aids, rather than replacing the driver wholesale.
Similarly, AM has a raft of abilities that can support human markers with specific tasks.
How can Automated Marking benefit students?
If used strategically, it should be able to improve marking efficiency, reliability, and transparency, all of which ultimately benefits students.
Automated Marking is efficient
Let's examine these three goals starting with efficiency.
AM excels in grading short factual answers and Selected or Closed Response items such as multiple choice questions.
Because there is no level of sophistication in these, it can read and compare responses with mark schemes to see easily which requirements have been met.
Where AM would struggle to mark as consistently as a human is with more complex and nuanced open form responses that can come in many guises.
Relieving humans of the simpler tasks could free them up to focus on assessing open response items.
How reliable is Automated Marking?
Next, there's reliability.
Consistent marking and scoring are essential for any assessment.
Current systems effectively support this goal.
Senior examiners monitor markers and correct anomalies such as keying errors or deviations from the mark scheme.
AM could be used to target key areas for greater scrutiny.
Its ability to cluster thousands of marked responses for any specified item could make it easier to identify aberrations such as drifting off the mark scheme and human error.
Automated Marking as a tool for transparency
Finally, transparency. This is crucial.
Students want to know that their marking and grades are accurate and, in the case of an appeal, exam boards want to show that they’ve got it right first time.
Visually highlighting key features of the mark scheme and linking it to corresponding sections in a response could be relatively simple for auto-markers.
This would provide insight into how scores were assigned.
This transparency could be shared with students which hopefully will boost confidence in marking and reduce the demand for appeals.
The future of Automated Marking
So, what does all this mean for the future of AM?
Well, we've identified some key trust and accountability concerns to the point where we know AM’s benefits and limitations.
Now, we’re exploring and improving these three ways in which it might be used to the benefit of examiners and the existing system.
Having determined where we want to go with Automated Marking places us in an excellent position to progress and achieve that.
Read more on this subject:
AI: assessing performance claims
Adaptive testing: Tailoring the future of assessments
Online Exams – The Robots Are Coming!